Enhancing urban resilience through machine learning-supported flood risk assessment: integrating flood susceptibility with building function vulnerability
This study assesses urban building flood risk in Guangzhou, in China, by integrating flood susceptibility with building function vulnerability. Using a Random Forest (RF) model, it predicts flood susceptibility based on flood records, hydrological, topographical, and anthropogenic features. The Categorical Boosting (CatBoost) model identifies building functions using POI and AOI data. Results reveal significant spatial variations: central districts exhibit higher flood susceptibility, while peripheral areas remain less affected.
Over half of the buildings are moderately vulnerable, with only a small fraction highly vulnerable. Based on flood susceptibility and functional vulnerability, Guangzhou is classified into three district types: central urban (Type I), intermediate urban (Type II), and suburban/rural (Type III). The study underscores the need for tailored flood risk management strategies to address these disparities and mitigate climate change-induced water hazards.
Explore further
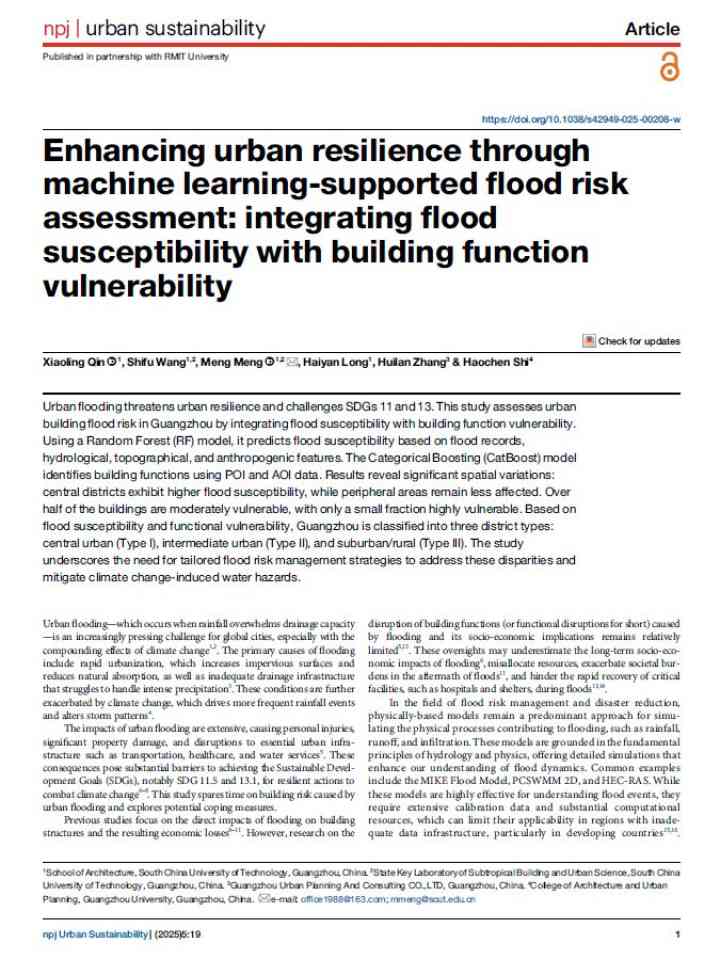