Scientists use AI to update data vegetation maps for improved wildfire forecasts
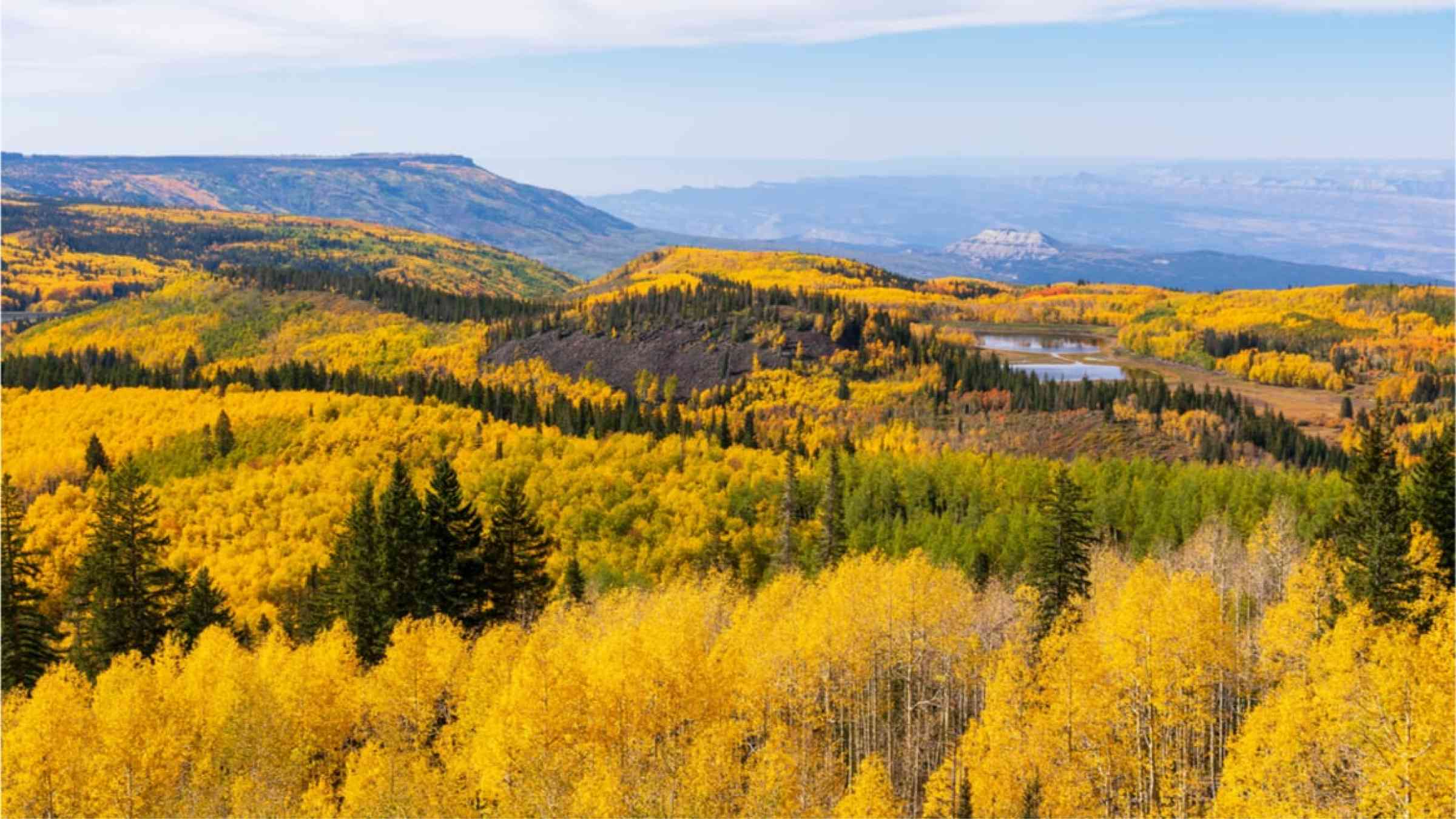
New technique substantially improved simulations of Colorado’s East Troublesome Fire
A new technique developed by the National Center for Atmospheric Research (NCAR) uses artificial intelligence to efficiently update the vegetation maps that are relied on by wildfire computer models to accurately predict fire behavior and spread.
In a recent study, scientists demonstrated the method using the 2020 East Troublesome Fire in Colorado, which burned through land that was mischaracterized in fuel inventories as being healthy forest. In fact the fire, which grew explosively, scorched a landscape that had recently been ravaged by pine beetles and windstorms, leaving significant swaths of dead and downed timber.
The research team compared simulations of the fire generated by a state-of-the-art wildfire behavior model developed at NCAR using both the standard fuel inventory for the area and one that was updated with artificial intelligence (AI). The simulations that used the AI-updated fuels did a significantly better job of predicting the area burned by the fire, which ultimately grew to more than 190,000 acres of land on both sides of the continental divide.
“One of our main challenges in wildfire modeling has been to get accurate input, including fuel data,” said NCAR scientist and lead author Amy DeCastro. “In this study, we show that the combined use of machine learning and satellite imagery provides a viable solution.”
The research was funded by the U.S. National Science Foundation, which is NCAR’s sponsor. The modeling simulations were run at the NCAR-Wyoming Supercomputing Center on the Cheyenne system.
Using satellites to account for pine beetle damage
For a model to accurately simulate a wildfire, it requires detailed information about the current conditions. This includes the local weather and terrain as well as the characteristics of the plant matter that provides fuel for the flames — what’s actually available to burn and what condition it’s in. Is it dead or alive? Is it moist or dry? What type of vegetation is it? How much is there? How deep is the fuel layered on the ground?
The gold standard of fuel datasets is produced by LANDFIRE, a federal program that produces a number of geospatial datasets including information on wildfire fuels. The process of creating these wildfire fuel datasets is extensive and incorporates satellite imagery, landscape simulation, and information collected in person during surveys. However, the amount of resources necessary to produce them means that, practically speaking, they cannot be updated frequently, and disturbance events in the forest — including wildfires, insect infestations, and development — can radically alter the available fuels in the meantime.
In the case of the East Troublesome Fire, which began in Grand County, Colorado, and burned east into Rocky Mountain National Park, the most recent LANDFIRE fuel dataset was released in 2016. In the intervening four years, the pine beetles had caused widespread tree mortality in the area.
To update the fuel dataset, the researchers turned to the Sentinel satellites, which are part of the European Space Agency’s Copernicus program. Sentinel-1 provides information about surface texture, which can be used to identify vegetation type. (Grass has a very different texture than trees, for example.) And Sentinel-2 provides information that can be used to infer the plant’s health from its greenness. The scientists fed the satellite data into a machine learning model known as a “random forest” that they had trained on the U.S. Forest Service’s Insect and Disease Detection Survey. The survey is conducted annually by trained staff who estimate tree mortality from the air.
The result was that the machine learning model was able to accurately update the LANDFIRE fuel data, turning the majority of the fuels categorized as “timber litter” or “timber understory” to “slash blowdown,” the designation used for forested regions with heavy tree mortality.
“The LANDFIRE data is super valuable and provides a reliable platform to build on,” DeCastro said. “Artificial intelligence proved to be an effective tool for updating the data in a less resource-intensive manner.”
Positioned for a positive impact
To test the effect the updated fuel inventory would have on wildfire simulation, the scientists used a version of NCAR’s Weather Research and Forecasting model, known as WRF-Fire, which was specifically developed to simulate wildfire behavior.
When WRF-Fire was used to simulate the East Troublesome Fire using the unadjusted LANDFIRE fuel dataset it substantially underpredicted the amount of area the fire would burn. When the model was run again with the adjusted dataset, it was able to predict the area burned with a much greater degree of accuracy, indicating that the dead and downed timber helped fuel the fire’s spread much more so than if the trees had still been alive.
For now, the machine learning model is designed to update an existing fuel map, and it can do the job quickly (in a matter of minutes). But the success of the project also shows the promise of using a machine learning system to begin regularly producing and updating fuel maps from scratch over large regions at risk from wildfires.
The new research at NCAR is part of a larger trend of investigating possible AI applications for wildfire, including efforts to use AI to more quickly estimate fire perimeters. NCAR researchers are also hopeful that machine learning may be able to help solve other persistent challenges for wildfire behavior modeling. For example, machine learning may be able to improve our ability to predict the properties of the embers generated by a fire (how big, how hot, and how dense) as well as the likelihood that those embers could cause spot fires.
“We have so much technology and so much computing power and so many resources at our fingertips to solve these issues and keep people safe,” said NCAR scientist Timothy Juliano, a study co-author. “We’re well positioned to make a positive impact; we just need to keep working on it.”