The benefits of better ocean weather forecasting
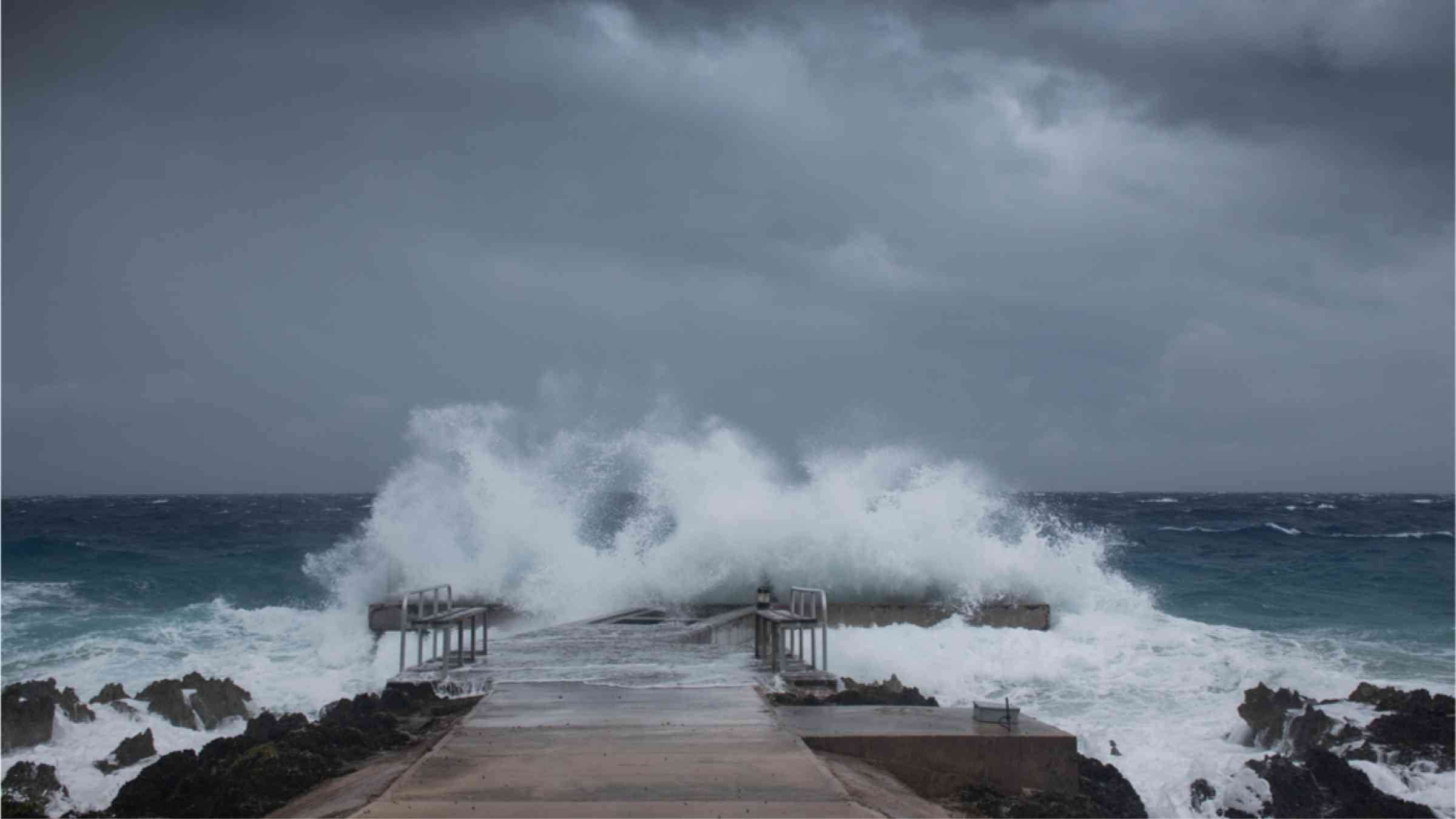
Improvements in our ability to forecast oceanic conditions weeks to months in advance will help communities, industries, and other groups prepare amid a changing climate.
Like atmospheric variability, variability in ocean conditions, such as sea surface temperature and salinity and sea ice cover and thickness, can have major effects on human activities and ecosystems both at sea and on land. For example, this variability, also called “ocean weather,” can influence the occurrence of fair-weather coastal flooding, which disrupts transportation and degrades coastal infrastructure around the world. Meanwhile, sea ice movement in an increasingly ice-free Arctic Ocean poses risks to high-latitude shipping. Elsewhere, changes in ocean upwelling can enhance coastal commercial fishing activity, whereas marine heat waves can disrupt fisheries and coral communities. As with atmospheric weather forecasts, knowing about ocean weather in advance can help minimize disruptions to everyday public and commercial activity, keep coastal and maritime workers safe, and aid marine conservation efforts.
Unlike atmospheric weather, however, which evolves on daily timescales, ocean weather typically evolves on weekly to monthly timescales (Figure 1). Slowly varying ocean conditions can regulate atmospheric weather, so including ocean feedbacks in atmospheric forecasting models can extend these models’ predictive skill by several days. Ongoing research is continually improving representations of ocean initial states (conditions at the start of a model calculation) and processes in coupled ocean-atmosphere forecast models. As a result, they have the potential to forecast ocean weather accurately weeks in advance—on subseasonal to seasonal (S2S) timescales—and provide needed guidance to stakeholders in communities and in marine and coastal industries, as well as to scientists, conservationists, and others.
Requirements of ocean weather forecasts vary depending on what information a particular stakeholder needs for operational decisionmaking. Fully understanding the benefits of S2S ocean forecasts to society requires an evaluation of the skill of these forecasts in the contexts of these informational needs (Figure 1). These evaluations will also fundamentally improve S2S forecasts overall by advancing knowledge of how initial conditions in an ocean model and ocean model physics affect forecast skill.
Here we describe recent advances in the science of ocean weather forecasting and walk through examples in which its application is increasingly important as the planet’s climate changes and human demands on Earth system services grow.
The Makings of Skillful Forecast Models
Most forecast models used for S2S prediction include sophisticated mathematical representations of the ways that ocean and sea ice states evolve over time. Including these processes allows for more realistic forecasts of air-sea interactions and coupled feedbacks than are possible with atmosphere-only forecasts. For example, coupling the ocean and the atmosphere makes it possible to construct forecasts of well-resolved and predictable ocean dynamics, such as equatorial Kelvin waves, which are an important part of the El Niño–Southern Oscillation (ENSO). Furthermore, the ocean provides “memory” to the climate system because it evolves much more slowly than the atmosphere and regulates fast varying atmospheric weather throughout the weeks-long forecast period. This memory can improve S2S forecasts of extreme winds, precipitation, or heat (Figure 1).
The predictability of ocean variables at S2S timescales depends on the underlying dynamic processes that drive ocean variability. For example, Figure 2 shows the predictability of three different variables—the strength of winds 10 meters above the surface, the depth of the ocean mixed layer (i.e., how deep turbulent water mixing extends), and sea surface height—averaged over the tropics in the European Centre for Medium-Range Weather Forecasts model. Mixed layer depth is tightly coupled to 10-meter winds, which is reflected in the very similar evolution of forecast skill with lead time for these variables. In contrast, the useful predictability of sea surface height has a long “tail” out to and beyond 6 weeks. This extended predictability window occurs because anomalies in ocean heat content, integrated over a range of depths, tend to persist over S2S time spans and because the dynamics of large-scale equatorial waves, which affect sea surface height, are highly predictable.
To improve understanding of ocean processes and aid development of ocean–sea ice forecast products, the World Weather Research Programme (WWRP) and World Climate Research Programme (WCRP) S2S Prediction Project database [Vitart et al., 2017] now provides multiple years of daily reforecasts (present-day forecasts of past events) and near-real-time forecasts from several operational models of various ocean and sea ice variables at 1° spatial resolution. These variables include sea surface temperature, height, and salinity; speed and direction of surface ocean currents; ocean mixed layer depth; mean potential temperature and mean salinity in the upper 300 meters; the depth of the 20°C isotherm (a proxy for the depth of the thermocline); and sea ice cover and thickness.
These reforecast data present opportunities for a broad assessment of model predictive skill for ocean or sea ice variables in S2S forecasts. Topics for future investigation and evaluation in this assessment could include systematic errors in forecast models (e.g., mean and variance biases, misrepresentation of key spatiotemporal patterns and physical mechanisms), ocean and sea ice initial conditions and their relation to atmospheric forecast errors, and areas of needed improvement in coupled forecast models (e.g., atmospheric-ocean feedback mechanisms).
The Growing Nuisance of Tidal Flooding
The skillfulness and forecasting abilities of ocean-atmosphere coupled forecast models are relevant for many applications, not all of which are far out at sea. As global temperatures and sea levels have risen, coastal communities have experienced more frequent nuisance, or fair-weather, tidal flooding. Locally, these events can disrupt traffic, inundate sewer systems, and damage property.
Nuisance flooding wreaks ecological damage as well. For instance, saltwater inundations of freshwater marshes in the lower Mississippi River delta hinder restoration efforts and stress native vegetation and ecosystems [Hiatt et al., 2019]. Such flooding also poses threats to coastal wildlife, including to nesting sites of endangered loggerhead turtles [Lyons et al., 2020].
Reliable S2S forecasts of nuisance flooding may benefit municipalities and conservation efforts alike. Municipalities can take action to mitigate damage and disruptions by, for example, preemptively distributing floodwall materials and developing plans to manage drainage systems. Loggerhead turtle conservationists can evaluate threats to existing nests by running “nest washover” models using output from S2S forecasts. They can then put volunteers on alert about the potential need to relocate nests to higher ground should a flood event be likely.
So far, the usefulness of S2S ocean weather forecasts for coastal flooding applications has been assessed to only a limited extent. However, a few recent studies have suggested that the frequency of localized nuisance flooding is regulated by large-scale climate phenomena like ENSO and the North Atlantic Oscillation. These and other large-scale climate indices are sources of S2S predictability of various atmospheric phenomena—such as wind circulation patterns and rainfall on the U.S. West Coast—and their apparent regulation of coastal sea surface heights suggests that they may regulate predictive skill for ocean weather as well.
A Changing Seascape for Arctic Shipping
For some industries, rising global temperatures pose both challenges and new opportunities. In the Arctic, fisheries; tourism; and the oil, gas, and mining industries all rely on offshore shipping. As the Arctic warms, thawing permafrost will reduce the availability of unpaved land-based transportation routes and further increase demand for ship-based transport by vessels that with less sea ice, do not require as much fortification and are thus less expensive [Stephenson et al., 2011]. However, reduced risks associated with sea ice loss may be partially offset by greater risks from increasing wave heights as Arctic winds drive wave activity over growing fetches of ice-free ocean.
Forecasts of sea ice concentration and sea state are essential for planning safe and efficient Arctic voyages. The three primary Arctic shipping passages—the Northern Sea Route, Arctic Bridge, and North Pole routes—are becoming increasingly accessible from July through September [Stephenson et al., 2011]. With passage times averaging 11–16 days, forecasts needed to plan voyages fall squarely within the S2S timescale.
Accurate ocean forecasts may be especially critical during the shoulder seasons when sea ice is forming or breaking up for the safety of both vessels and whales that migrate to and from the Arctic during these periods. Increased shipping activity poses multiple risks to whales, including a greater likelihood of ship strikes as well as underwater noise pollution, which disrupts communication within whale pods [Hauser et al., 2018].
Some current global forecast models are already able to provide reliable sea ice concentration forecasts for the Arctic shipping season. Zampieri et al. [2018] analyzed Arctic sea ice concentration predictions by models contributing to the WWRP-WCRP S2S database and found that several could skillfully forecast sea ice concentration as much as 2–6 weeks in advance. Such studies highlight the potential to further improve sea ice forecasts by incorporating better observations (and thus model initial conditions) of ocean salinity, heat content, mixed layer depth, and currents.
Reliable forecasts of these variables on S2S timescales could also translate into more confident estimates of the distributions of Arctic marine life based on the forecasted ocean state. Quantifying model forecast skill for these ocean variables through analysis of the S2S database may thus contribute to preserving Arctic ecosystems while simultaneously helping to ensure the safety of human visitors.
Better sea ice forecasts are also needed to keep pace with increasing interest in monitoring and operating in the ocean around Antarctica, driven by, for example, climate-focused research and conservation efforts (e.g., enhanced marine protected area coverage). Planning for efficient supply deliveries to multiple research bases around Antarctica and for reducing risks to vessels requires accurate subseasonal forecasts of sea ice variables (e.g., ice age and movement, snow cover, fast ice formation and breakup) and of the surrounding sea state (e.g., wave heights). Although the number of vessel users will likely remain low in this region, the remoteness and the challenging conditions increase the hazard of adverse weather.
Fisheries and Marine Management
Seasonal and subseasonal forecasts of ocean temperature are important tools in managing marine ecosystems. Salmon aquaculture operations adjust their harvest and feed schedules in response to warnings of a warmer than normal summer [Hobday et al., 2016]. Fisheries use these forecasts to plan port departures to maximize ships’ catches while minimizing time spent at sea. Fishing regulatory bodies use subseasonal to seasonal ocean temperature forecasts to model species habitat distributions—of endangered southern bluefin tuna, for example—which inform fishery zoning as well as other marine industries [Tommasi et al., 2017]. Coral reef managers on the Great Barrier Reef have been using multiweek to seasonal forecasts of ocean temperature and thermal stress for more than a decade as part of their early-warning system for coral bleaching [Smith and Spillman, 2019].
Marine industries, in addition to needing to cope with warmer than usual seasonal conditions, are increasingly concerned about shorter-duration marine heat waves, which can occur at any time of year. These extreme events have had devastating impacts on marine ecosystems in recent years [Holbrook et al., 2020]. For example, in 2014, the “Blob,” a large expanse of unusually warm water in the northeastern Pacific, caused toxic algal blooms that closed crab and shellfish industries for months. The 2016 Great Barrier Reef marine heat wave caused severe coral bleaching and mortality, and a heat wave in the Tasman Sea the same year affected salmon aquaculture in southeastern Australia.
Prediction of these events at subseasonal timescales would allow marine industries and managers to fine-tune operational plans and implement strategies to minimize impacts on their businesses and on resources. Data sets from the S2S Prediction Project will help facilitate investigations into the drivers of extreme ocean temperatures as well as experiments to explore their predictability and improve capabilities to forecast these conditions on daily to weekly timescales.
Scientists must communicate the degree of accuracy and skill of model forecasts when they engage with fisheries, reef, and aquaculture managers so that these stakeholders understand the basis for and the degree of confidence in predicted outcomes before they apply the predictions in their operational decisionmaking [Hobday et al., 2016]. The S2S Prediction Project offers opportunities to assess different S2S models (see sidebar), their sources of forecast skill, and other strengths and weaknesses for different applications. Such assessments (e.g., Figure 3) will be invaluable for improving ocean forecasts and increasing the confidence in and uptake of forecast tools by marine stakeholders.
Tools for Analyzing S2S Ocean Model Outputs
Since January 2020, five of the 12 models in the S2S Prediction Project have been providing forecasts of the ocean and sea ice variables noted in this article. The archive of these forecast data, hosted at three archiving centers, represents an extensive and important resource for research on subseasonal ocean predictability and ocean weather impacts.
The International Research Institute for Climate and Society (IRI) has been supporting partners around the world to codesign, implement, produce, and verify forecasts at multiple timescales, a general approach commonly referred to as NextGen. As part of the NextGen methodology, IRI has developed PyCPT[Muñoz et al., 2019], a set of Python language libraries that interfaces with IRI’s Climate Predictability Tool (CPT; Figure 3).
These tools help with the following tasks:
• analyzing sources of predictability of atmosphere and ocean variables
• conducting deterministic and probabilistic predictive skill assessments and verifications
• performing simple and pattern-based statistical model calibration and downscaling
• building multimodel ensembles using a variety of techniques
• operationalizing calibrated multimodel ensemble forecasts at subseasonal and other timescales
PyCPT can automatically download the required model and observational data sets for the region and dates of interest directly from the IRI Data Library, simplifying these tasks.
A Bridge to Earth Science Careers
As the growing global population increases demands on climate services—everything from food security to energy to health monitoring and prediction services—so too increases the need for a workforce trained to understand how the physics of (and variability in) Earth system processes can significantly affect the well-being of humans and ecosystems and to adapt to new challenges. Imbuing undergraduate STEM (science, technology, engineering, and mathematics) education with discussions of issues that are societally relevant and of interest to students—such as conservation, climate, and urban planning—can increase students’ exposure to and interest in geosciences in personally meaningful ways [Teasdale et al., 2018].
As illustrated above, S2S ocean forecasts are important in addressing many challenges and for many different needs, from model development by researchers to various commercial activities, public safety, and conservation efforts. One way to help develop a workforce trained to meet these challenges is to introduce undergraduate students to the idea that improving these forecasts depends critically upon advancing our understanding and representation of the physical processes predicted by forecasting models. The study of ocean weather, its predictability on S2S timescales, and its impacts can thus serve as a bridge to science understanding and a gateway to Earth science careers for students interested in solving societally and environmentally relevant problems.
By sustaining a well-trained and motivated workforce and by continuing to study and improve the modeling of ocean variables, we can better assess how ocean weather predictions benefit a range of stakeholders and further develop the reliability of forecasts weeks to months into the future to help protect infrastructure, manage resources, and promote safety for workers and the environment.