Monitoring earthquakes at the speed of light
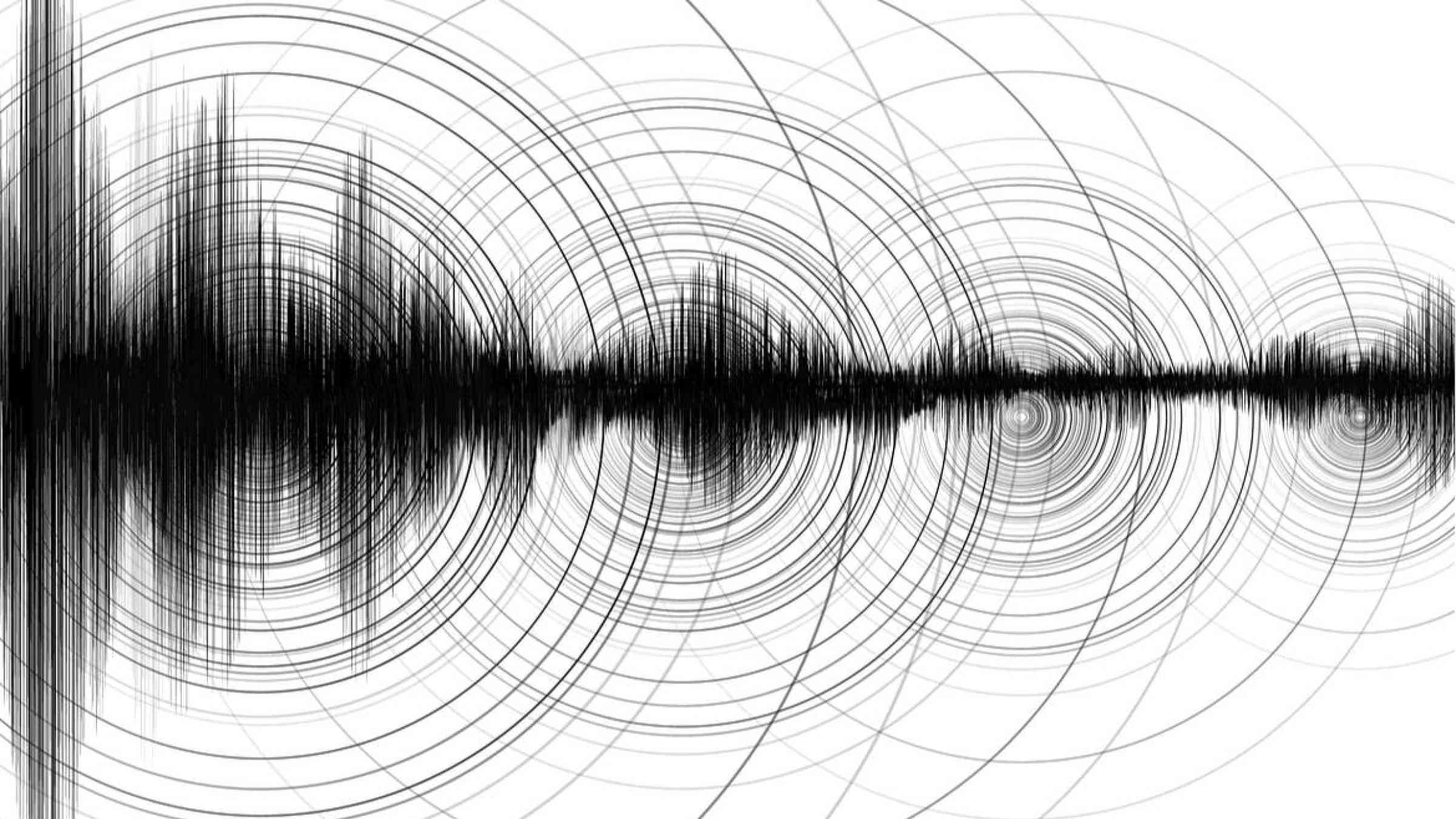
Earthquakes and the tsunamis they generate have caused almost a million casualties in the past 30 years. Many alert systems have been developed to limit the human and material costs of these natural disasters. However, these systems have difficulties in estimating quickly and accurately the magnitude of very large earthquakes.
Now, a study published in Nature has described a machine learning model that recognizes patterns in seismic data to better estimate the magnitude and location of a large earthquake.
Using 350,000 modeling scenarios of earthquakes initiating at 1,400 potential earthquake locations in Japan, Andrea Licciardi, a geophysicist at the Université Côte d’Azur in France, and his colleagues succeed in instantaneously estimating the magnitude of large earthquakes on the basis of prompt elastogravity signals (PEGS).
PEGS are gravitational perturbations generated by the motion of large masses of rocks during an earthquake. They propagate at the speed of light, carrying earthquake information much faster than seismic waves traditionally used in early-warning systems.
Scientists have known that although in principle PEGS could help speed up earthquake warnings, their very weak amplitude has prevented their use in alert systems. The researchers in the new study overcame this limitation thanks to an artificial intelligence algorithm based on Global Navigation Satellite System data. Using the algorithm, they showed that the magnitude of large earthquakes could be accurately estimated on the basis of PEGS seconds after the earthquake starts and tracked as the earthquake grows.
“I believe that this paper is interesting. Actually, the discovery of PEGS was already done in my paper of 2016 and then confirmed in the paper of Vallée et al. in 2017,” said Jean-Paul Montagner, a geoscientist at the Institut de Physique du Globe de Paris who was not involved in the new research. “So what is innovative in this paper is the use of machine learning techniques, which makes it possible to improve the detection of these very small signals.”
Licciardi agreed. “The main advantage of our model relies on the underlying data, the elastogravity signals,” he explained. “Once an earthquake occurs, these signals travel faster than seismic waves and are strongly sensitive to the earthquake magnitude.…Because of that, our model can estimate the magnitude of the earthquake faster and more accurately than conventional early-warning systems based on P waves at least for large earthquakes (magnitude above 8.3/8.4).”
Improving Tsunami Early Warning
Licciardi pointed out that the model’s response time of about a minute can dramatically improve tsunami early-warning forecasts. In a real-time scenario, he said, the magnitude retrieved by the model can be used to quickly estimate the size of the induced tsunami wave and therefore mitigate its impact.
“Classical early-warning systems based on P waves can’t distinguish between a magnitude 8 and a magnitude 9 earthquake, while our model does not suffer from this limitation,” said Licciardi. “It provides the most accurate estimation of magnitude as a function of time.”
The new model’s strength in forecasting large earthquakes “is because the elastogravity signal is strongly sensitive to the magnitude of such large earthquakes,” Licciardi explained. “In fact, the applicability of our model is limited to such large earthquakes (magnitude above 8.3/8.4) because the signal’s amplitude for relatively smaller earthquakes is too small and buried in the background noise. This is why other tools and data are still needed in the context of early warning.”
“This is important for earthquake early-warning systems because for the largest earthquakes there is an extended time (up to minutes) in which they gather magnitude and strength,” explained Andreas Plesch, a senior Earth scientist at Harvard University who was not involved in the new work. “The authors correctly point out that during this extended time the method, especially if combined with other methods, has the operational potential to track the growth of such an earthquake earlier and more accurately.”
Plesch further noted that using the new model, tsunami alerts could be issued not only earlier (by tens of seconds or perhaps even minutes) but also more confidently and with better wave height estimates derived from improved magnitude estimates.