Inclusive integration of artificial intelligence in DRR
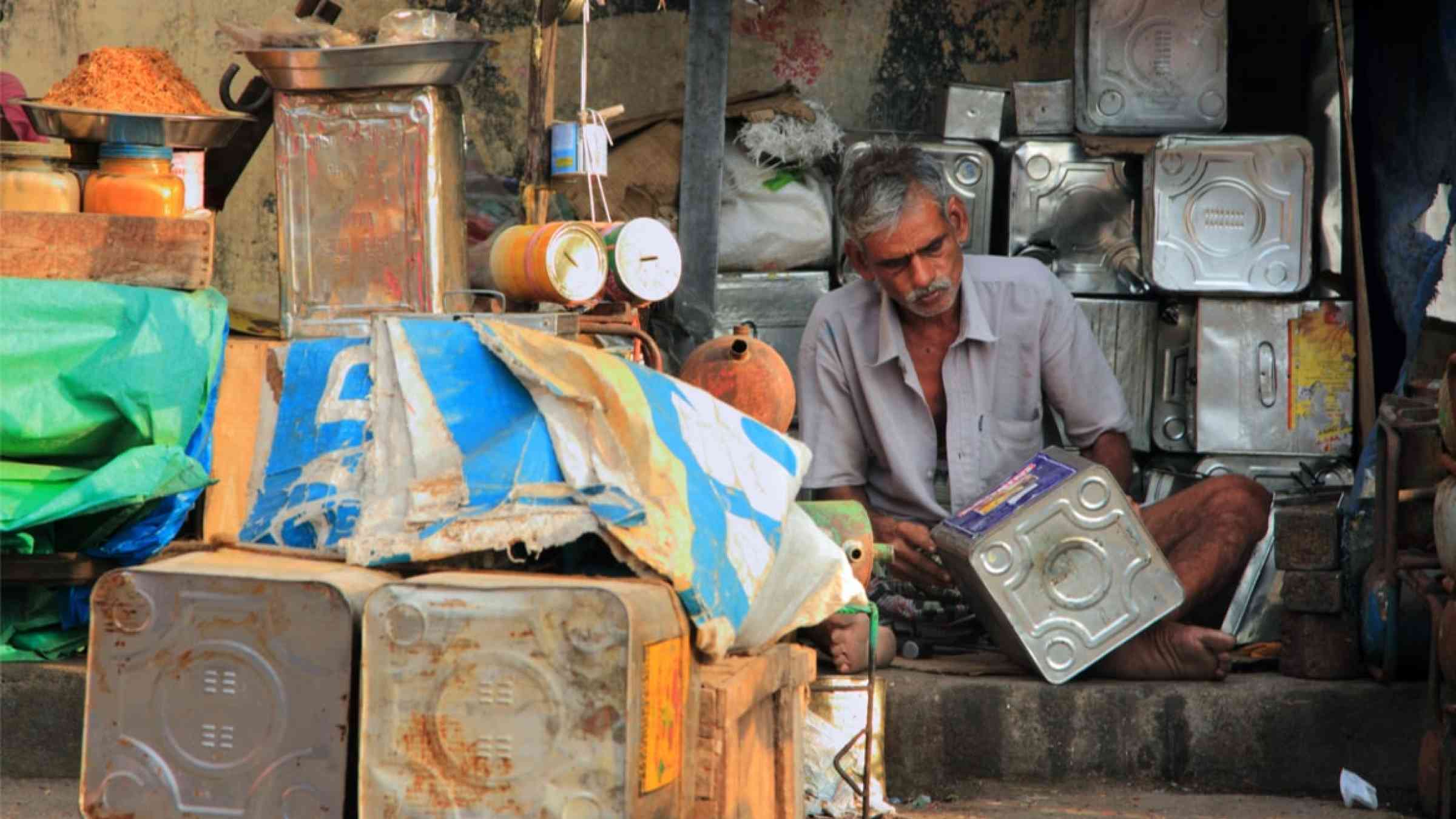
Disasters often expose society's most profound vulnerabilities. While efforts to mitigate these challenges are ongoing, the incorporation of artificial intelligence (AI) into disaster risk reduction (DRR) presents both opportunities and threats.
Central to this discussion is a crucial question: How do we ensure AI's application in disaster management neither harms nor neglects marginalised groups? This question goes beyond mere technical aspects, touching upon socio-political dimensions.
AI has the potential to revolutionise disaster prediction, response, and recovery. It can quickly analyse vast amounts of data to forecast weather events, evaluate damage, and distribute resources effectively. Yet, if AI models are trained on biased or non-representative data, they may produce misleading results. Such inaccuracies could exacerbate existing inequalities and further sideline groups already at a disadvantage.
Marginalised communities are often disproportionately affected by disasters, not because of inherent vulnerabilities but due to societal disparities. If DRR strategies, enhanced by AI, fail to consider this, the technology could unwittingly cause more harm than good.
In this exploration, we delve into AI's role in DRR, its implications for marginalised groups, and ways to leverage AI inclusively, ensuring social justice for everyone.
Impact on marginalised groups
Understanding AI's role in DRR necessitates highlighting the challenges confronting society's most vulnerable. The experiences and concerns of diverse marginalised groups remind us that technological advancements should align with a commitment to equity and representation. Only then can AI truly benefit those most at risk during disasters.
For individuals with disabilities, daily life poses numerous challenges, even without the threat of disasters. Introducing AI into DRR policy could inadvertently compound these difficulties. A key issue is the nature of data on which AI systems are trained. If AI predominantly uses data from able-bodied individuals, or neglects the specific needs of those with disabilities, its suggestions or influenced systems may miss or misinterpret essential needs for these individuals.
For instance, AI-informed disaster evacuation plans may neglect to consider accessible routes for those with mobility issues. AI-generated early warning systems might rely heavily on sound or visual signals, failing those with hearing or visual impairments. Without comprehensive and representative data, DRR strategies might be technologically advanced but remain ill-equipped to protect the most vulnerable. Thus, it's not just a technical need, but a moral obligation, to ensure AI models in DRR consider everyone, regardless of their abilities.
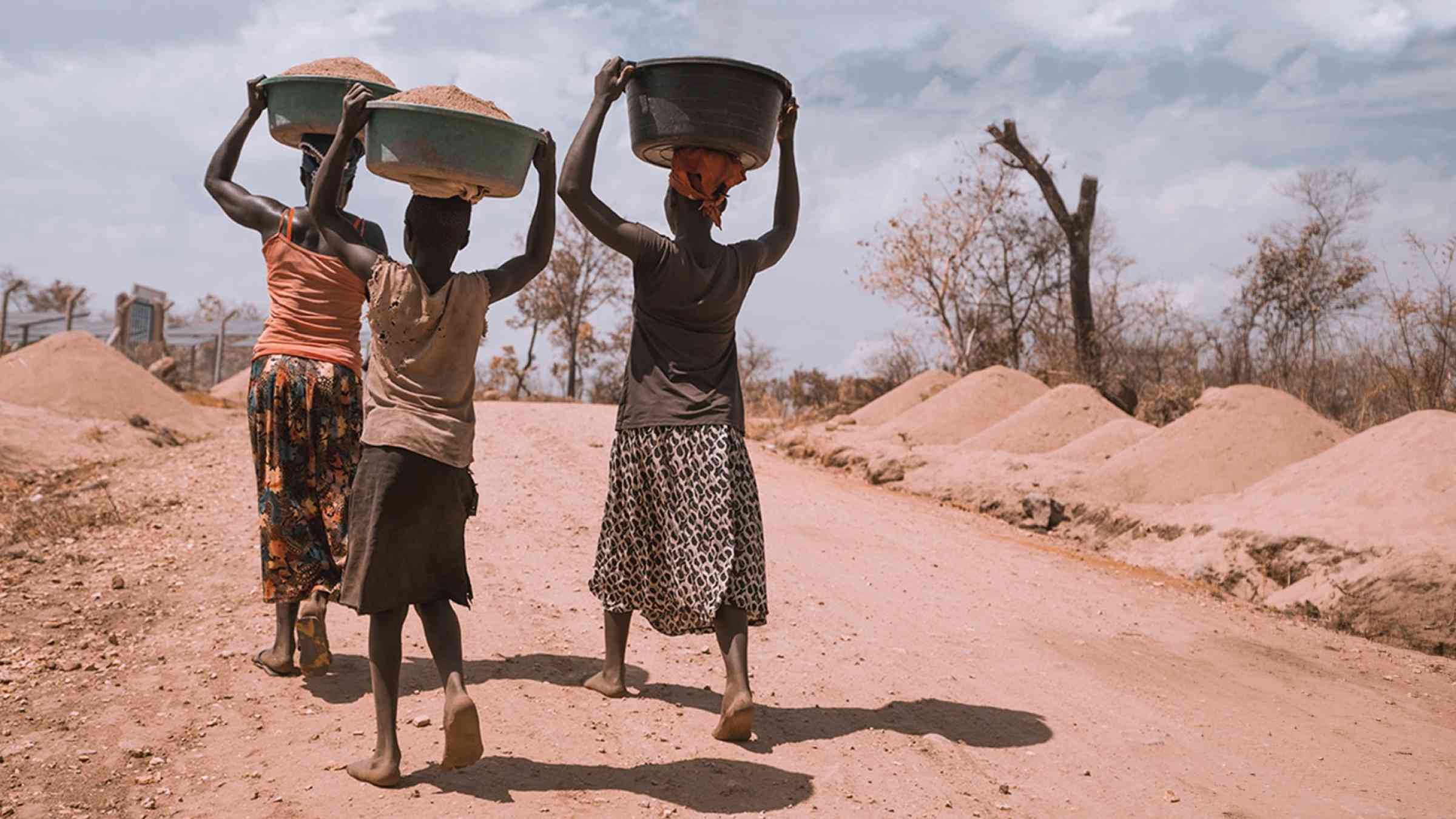
Refugees and migrants, displaced due to conflict, economic struggles, or environmental crises, face unique vulnerabilities. As countries increasingly employ AI in DRR policy, these populations might be overlooked or misunderstood. Their movements are often unpredictable, and AI systems not trained on diverse data might misjudge their dynamics, leading to ill-preparedness or insufficient resources in regions temporarily housing these groups.
Additionally, refugees and migrants might not be adequately represented in AI training data, resulting in DRR strategies that overlook their linguistic, cultural, or trauma-informed needs. This highlights the importance of ensuring DRR technologies are inclusive, adaptable, and sensitive to the challenges each marginalised group faces.
Homeless or transient communities, like the Roma, confront unique vulnerabilities during disasters. If AI systems used in DRR policy don't consider their nuances, these vulnerabilities could increase. Most AI models for DRR are based on data from mainstream or settled communities, often neglecting or misrepresenting transient groups. This oversight could lead to misplaced aid or ineffective early warning systems. Recognizing and accommodating the unique cultures and lifestyles of such groups is essential to ensure that DRR strategies are not only effective but also inclusive and sensitive.
Those in the informal economy, while significant in many urban landscapes, often lack representation in formal datasets. This invisibility becomes problematic when AI is incorporated into DRR policies. Since their work often requires discretion and operates under unique conditions, conventional datasets may fail to capture their vulnerabilities. Such oversight could result in ineffective disaster preparedness and response strategies for these groups.
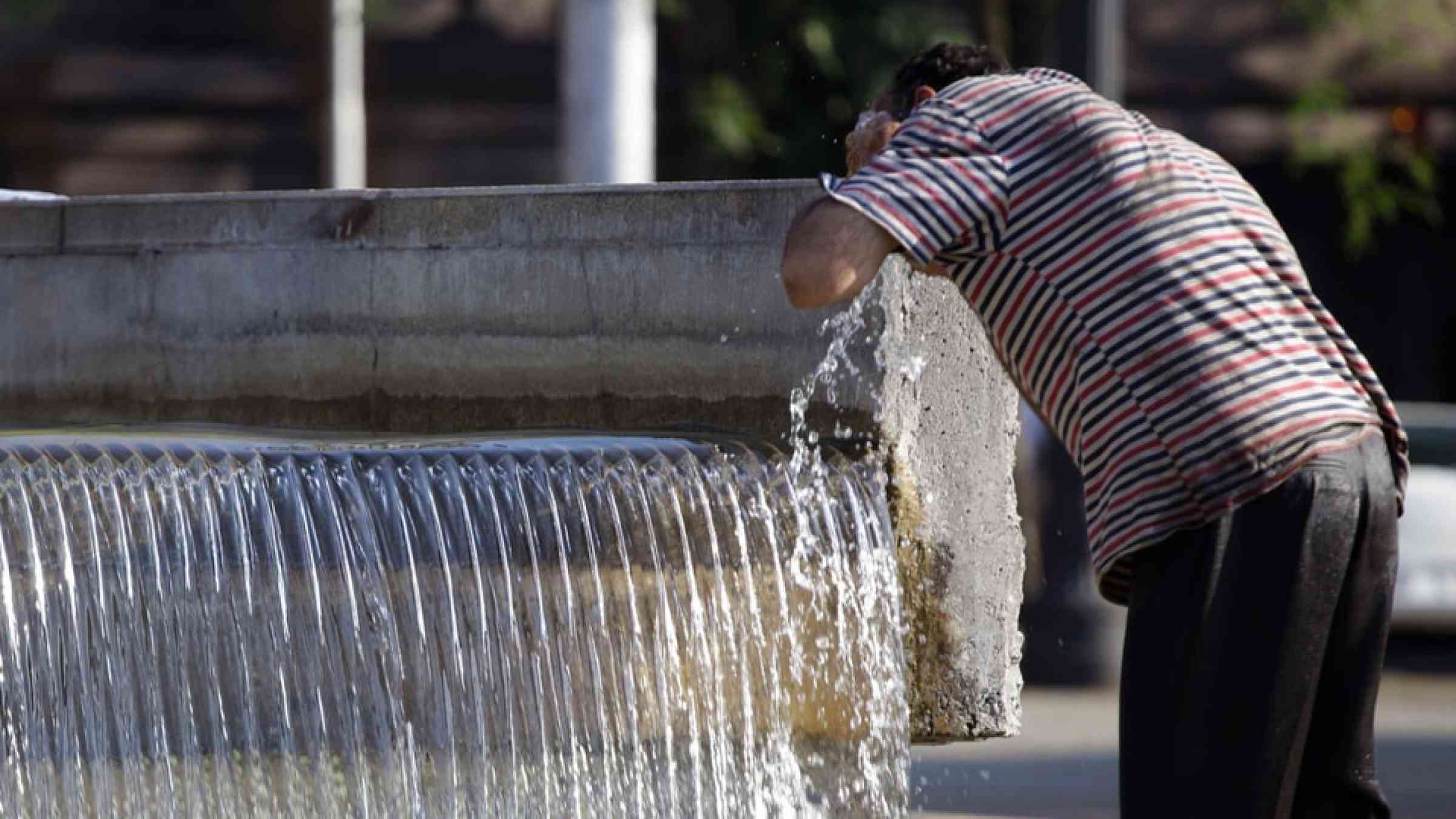
Recommendations
To ensure the integration of AI in DRR is inclusive and equitable, the following recommendations are proposed:
Inclusive data collection and training
Prioritise the inclusion of all societal segments, especially marginalised groups, in the datasets used for training AI systems. Collaborate with diverse sources, like NGOs and community leaders, for comprehensive data collection.
Cultural and contextual sensitivity integration
Design AI systems that understand cultural, linguistic, and societal nuances, ensuring DRR strategies are both effective and inclusive.
Feedback and iterative improvements
Regularly update AI systems in DRR to reflect societal changes, incorporating feedback from marginalised communities to maintain relevance.
Legal and ethical safeguards
Establish frameworks that protect individual rights and privacy, especially for marginalised groups, ensuring AI's use in DRR is equitable.
Stakeholder collaboration and community engagement
Collaborate with community leaders and NGOs to develop AI-driven DRR strategies, ensuring they are grounded in reality and inspire trust.
AI's intersection with disaster risk reduction offers both potential and challenges, especially for marginalised groups. Misrepresentation, oversight, and unintentional harm arise mainly from non-representative data and cultural insensitivity. To harness AI's transformative power in DRR, a commitment to inclusivity, continuous feedback, and context-sensitive technology is essential. By prioritising the voices of the most vulnerable, AI can usher in a new era of equity, justice, and resilience in disaster management.